Fuelling data-driven UFT policies
Authored by Lukas Marthaler
- Introduction
With the rise of urbanization across the globe, increasingly more pressure is put on Urban Freight Transport (UFT) to provide the last-mile delivery of goods. Simultaneously, increased efforts should be and are made towards reducing the impact of UFT on the environment. Namely, UFT accounts for approximately 6% of global transport-related GHG emissions (Civitas, 2020). Moreover, global freight demand is expected to triple between 2015 and 2050 (Raj & Vigran, 2020).
Coping with these challenges can be complex for policy decision-makers due to the trade-offs between factors like accessibility for logistics service providers, liveability and provision of goods for citizens, and climate. There is a need for fact-based sustainable planning to deal with this increased complexity. By considering empirical data on Key Performing Indicators (KPI’s) of urban logistic systems, policymakers can, in theory, address one issue without worsening another. These are three out of many advantages associated to working with quantitative indicators for policy planning (Rai et al., 2017):
- The ability to capture complex phenomena into manageable and meaningful units
- The ability to facilitate comparison, benchmarking, and communication
- The ability to highlight trends
However, the past two decades have shown a barrier towards the increased demand for fact-based policies: data. In the recently published European Urban Mobility Framework, the need for data in fact-based decision-making is underwritten prominently. Detailed and fresh data streams are needed to fuel data-driven policies. However, gathering data proves to be complex, costly and time-consuming.
2. The data gap
Two types of data problems have surfaced (1) Currently available data sets are not comprehensive or specific enough. (2) Data acquisition is costly and time-consuming.
Currently available data not useful enough
Many urban freight data collections are general, averaged, and therefore unable to address specific urban issues (Rai et al., 2017). Other urban datasets containing macro-indicators (urban sprawl, city size and population density) also do not provide the necessary insights on their own, without overlaying the necessary freight-related data. Some examples of data gaps include (Allen et al., 2014):
- Activities of Light Goods Vehicles and Heavy Goods Vehicles are often not separated in data collection
- Data about logistics infrastructure from which urban freight deliveries depart is lacking
- The link and relationship between urban freight activity and freight activity further upstream in the supply chain is not well documented
Of the 45 quantitative UFT indicators formulated by Rai et al. (2017), 19 indicators (42%) could not be fulfilled with available data in their case study. This depicts the gap between necessary insights for sustainable UFT planning and the data available.
Current data acquisition is expensive and time-consuming
Today, data collection is done through a variety of collection methods, each with its own purpose, advantages, and disadvantages. These include surveys, interviews, group discussions, traffic counts, written questionnaires, diaries, and on-board technologies (Hadavi, 2018) . These methods are often costly because of the necessary human resources, and none provide a single comprehensive image. Moreover, these data collection methods can be complicated and thus time-consuming. For example, retrieving data from on-board technologies can be problematic since companies are not obliged to share the data.
3. Bridging the data gap:
Two directions are mainly explored by academics and EU-funded projects: (1) lowering costs and complexity of data collection through new technologies and (2) leveraging existing data sources through novel data-collection frameworks.
(1) Lower-cost data collection through technology application
Technology application in data collection for UFT planning has not reached the same level as traditional traffic counting and surveys. Technologies that have received most attention so far include loops, Automatic-Number Plate Recognition (ANPR), Bluetooth and floating car data (Hadavi, 2020). Applying such technologies could lower overall costs and be more scalable than manual counting or use of surveys (Raj & Vigran, 2020).
Most of these technologies, however, require an initial investment and require expertise to process and analyse the gathered raw data. Moreover, every technological application has its inherent drawbacks, which means there is no single silver bullet.
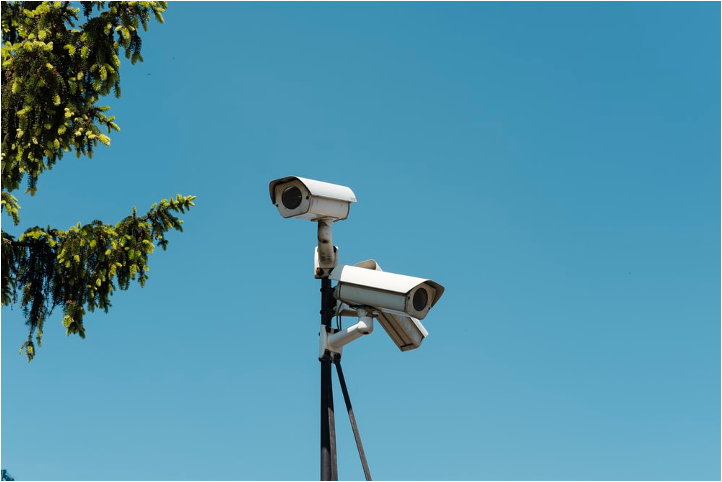
ANPR cameras
(2) Novel data-collection frameworks: Centralisation & structuring of available public data
Currently, UFT data is scattered across stakeholders and can take much effort to collect. Stakeholders like logistics service providers (LSP), local statistic bureaus, policy departments and national ministries hold different data sets which can be useful for fact-based decision-making. Examples of solutions and efforts towards this issue include local UFT observatories and development of data collection frameworks projects.
Supported by the Catalan government, the Cimalsa logistics observatory provides yearly updates on several UFT related KPI’s in Catalonia. They gather data from a large variety of sources including private stakeholders, data vendors and various public authorities. Although decision-makers might require more frequent updates for certain policy-testing purposes, this type of data can provide information for longer-term developments and decision-making.
In a related field, urban passenger mobility, the URBANITE project is putting effort behind automatic data collection and processing mechanisms. This works shows promising cross fertilization possibilities for UFT data collection, which could bring much value (Rai et al., 2017).
Within UFT, tools like the NOVELOG framework could form a starting point for data collection, as it provides four thematic pillars under which UFT data can be categorized. The framework also differentiates between different stages of data collection and interpretation.
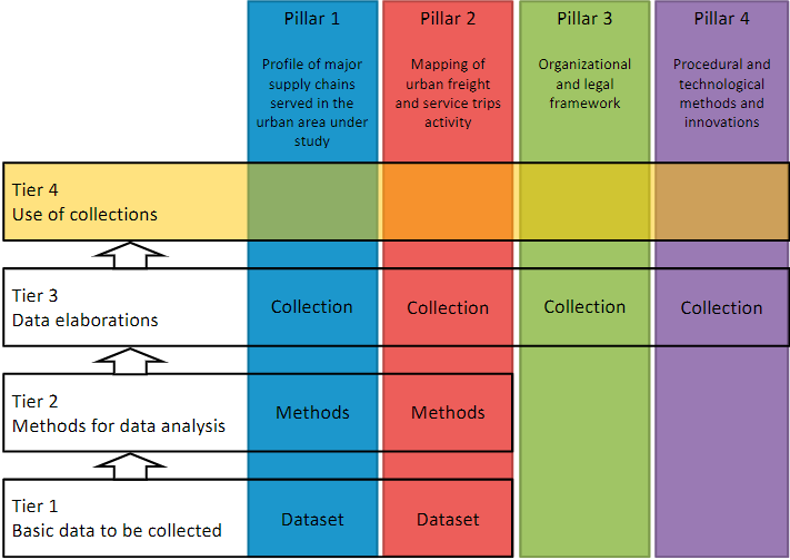
Here, the difficulty of obtaining data from logistics service providers should not be underestimated. Conflicting interests between city planners and LSP have shown to prohibit data flows between the two parties. Research among LSP’s by Laegran (2021) shows the following prerequisites they have for sharing data with public authorities:
- Sharing must benefit the carriers, in terms of better planning/facilitating etc.
- The goal of the data collection must be better city logistics, including improved distribution conditions for the carriers.
- Complying with the data sharing terms, ensuring data security.
- Anonymization and on aggregated level, complying with market considerations.
- “All” carriers contributing with data.
- Need to have defined what the data will be used to – a plan and purpose for the data, clear framework, and boundaries for the use.
- The data should be easy to access and extract from the carriers’ systems.
4. Conclusion
All in all, whereas data-based policy planning could have much potential in UFT, its primary resource, data, is still not delivered in full. Data is scattered across stakeholders, not comprehensive enough and costly to acquire. Here, solutions could be stimulated by the focus of the European Commission on data, as described in the recently published European Urban Mobility Framework.